Autonomous Greenhouse from Microsoft is up to the Challenge
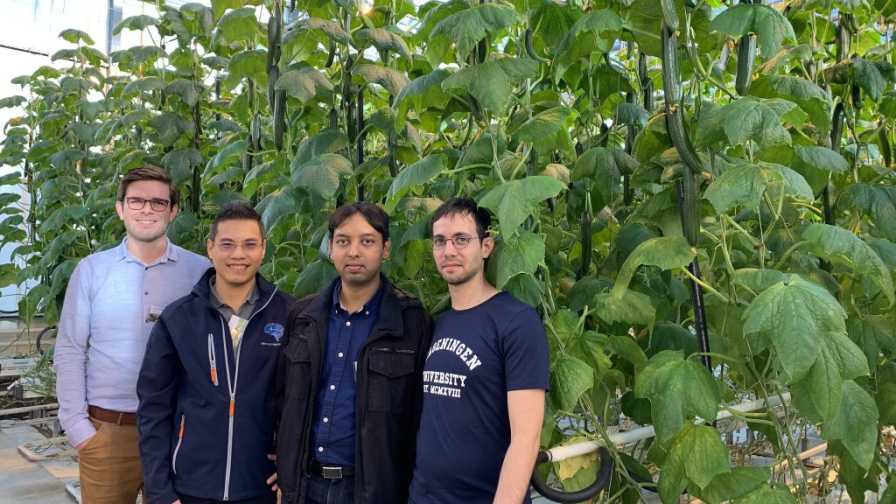
Microsoft Research’s Kenneth Tran (second from left) led Team Sonoma, which won the first International Autonomous Greenhouse Challenge by successfully growing greenhouse cucumbers in the Netherlands for four months while operating remotely from Redmond, WA.
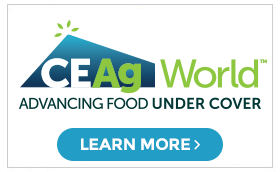
As revealed earlier this year at the 2019 PrecisionAg VISION Conference, the FarmBeats project from Microsoft’s research arm is targeting large-scale farming through components including its Azure cloud-computing service as well as data transmission through unallocated spaces of television frequencies.
MSR has an interesting parallel effort underway in specialty crops called Project Sonoma – charged with creating an autonomous greenhouse for vegetable production. The project is “very early stage,” Kenneth Tran, a Principal Research Engineer in the Reinforcement Learning Group of MSR, told attendees at the 2019 Canadian Greenhouse Conference in Niagara Falls, ON, Canada.
Yet the technology behind the project is being positioned for commercialization. “It’s fair to say that the tech is commerce ready (with some productization and marketing efforts),” he told me in an email. “However, as a researcher, I still see that there’s way more room for improvement, and we’d rather focus on it first.”
The project’s objective was straightforward if a little daunting: to develop “an algorithm that can operate indoor farms autonomously and efficiently – in both vertical farms and greenhouses,” Tran said. The team, he said, had two major questions: Can horticultural knowledge be encoded into an algorithm to automate decisions? And: Can A.I. outperform an experienced-human-operated greenhouse?
Those questions were put to the test when Microsoft competed in the International Autonomous Greenhouse Challenge, organized by Tencent in China and Wageningen University and Research (WUR) in the Netherlands and held for the first time late last year. (The contest is now in its second edition.)
Five teams from nine nations were invited to participate, each assigned 96 square meters (about 1,000 square feet) of greenhouse space at WUR to grow a cucumber crop. “Team Sonoma” entered the contest greenhouse one day before the competition started to set up web cameras and what Tran called “very typical sensors” – ventilation, heating, fogging, CO2, light, etc. – then operated the greenhouse remotely from Redmond, WA, U.S., for the next four months. Among the technologies the team deployed were pattern recognition (phenotyping and anomaly detection), computer vision (measuring the plant biomass, anomaly detection such as tip burn and healthy vs. unhealthy plants), and robotics (transplanting, harvesting). Also part of the tech set was machine learning and “black-box optimization.”
Each day advisor Xiuming Hao, a greenhouse production expert with Agriculture and Agri-Food Canada (AAFC), checked all of the crop’s data at the team’s online project site, reviewed webcam images, and suggested tweaks in the control settings. It was a combination of what Tran called “machine learning boosted by domain knowledge” – the latter the “rules-based process that growers use today.”
The result? Team Sonoma ousted all its competitors including teams from Tencent and Intel and two highly regarded Dutch greenhouse vegetable growers, Tran said. Their net profit on cucumbers was 17% higher than the next nearest competitor – the human growers – and bested the next nearest tech team by 23%.
Tran was understandably excited though also happily surprised and a bit humbled by Team Sonoma’s success. “I had not grown any plant or any tree in my life until this challenge,” he said. “We didn’t have high expectations. We just wanted to get our feet wet.”
Yet, Team Sonoma’s work continues. “What we have achieved is just a delta of what we can achieve next, we believe,” Tran said.